Artificial Intelligence, Machine Learning, Deep Learning
- hennag81
- Nov 11, 2024
- 5 min read
Updated: Nov 13, 2024
#ArtificialIntelligence #MachineLearning #DeepLearning #AIApplications #FutureOfAI #AICapabilities #AICategories #AIExamples #AIPredictions
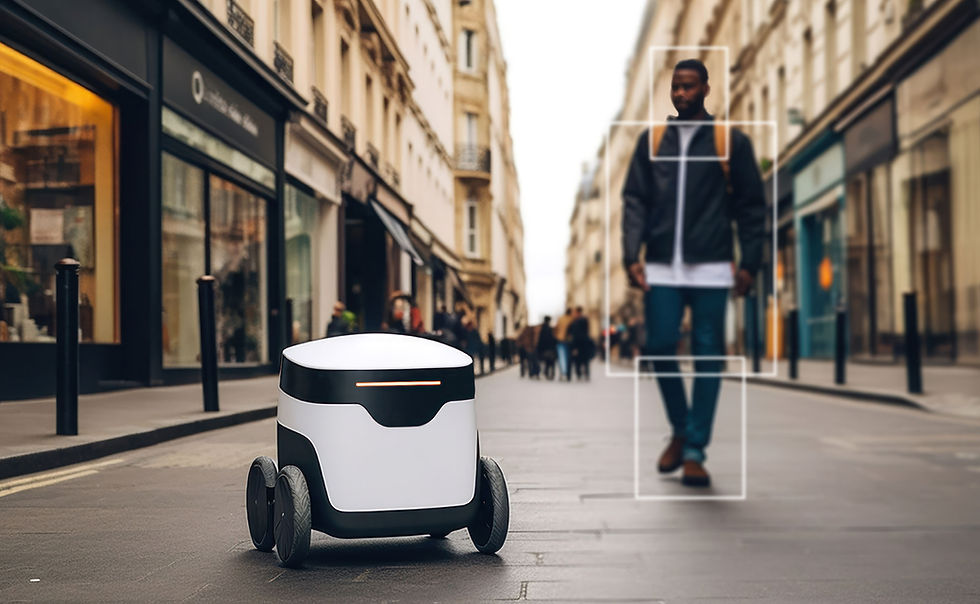
Artificial Intelligence (AI) has rapidly evolved from a concept in science fiction to a powerful influence in our everyday existence. From voice-activated virtual helpers to autonomous vehicles, AI is transforming sectors, altering human engagement, and improving ease of use. So, what precisely is AI, and in what way does it operate? This article examines the definition of AI, its applications, capabilities, various types, and the optimistic future it holds.
What do Artificial Intelligence, Machine Learning, Deep Learning mean?
Artificial Intelligence denotes the imitation of human intellect in machines crafted to think, learn, and address challenges. In contrast to conventional software, AI systems evaluate data, identify patterns, and make choices, consistently enhancing their performance as time goes on.
Essential Characteristics of AI:
Data Examination: AI employs extensive data sets to reach conclusions and make knowledgeable choices.
Education: Machines gain knowledge from their experiences and adjust to new data.
Adaptation: AI systems improve over time by acquiring additional data, enhancing their performance in particular tasks.
Uses of Artificial Intelligence
Artificial Intelligence, Machine Learning, Deep Learning have infiltrated various industries, providing sophisticated solutions that improve efficiency and enhance user experience. Here are several of its most significant uses:
Mobile Phones and Voice Assistants: Artificial Intelligence drives Siri, Google Assistant, and Alexa, allowing users to perform hands-free activities such as managing schedules, responding to questions, and operating smart home gadgets.
Automotive Sector: Autonomous vehicles and sophisticated driver-assistance technologies depend significantly on AI for routing, obstacle recognition, and instantaneous decision-making.
Social Media Networks: Customized feeds, focused ads, and facial recognition technologies improve user interaction and content distribution.
Video Gaming: AI enhances gaming through the development of adaptive, lifelike adversaries and ever-changing settings.
Banking and Finance: AI aids in identifying fraudulent activities, evaluating credit risks, and offering round-the-clock customer assistance via chatbots.
Healthcare and Monitoring: In the medical field, AI aids diagnostic instruments and robotic surgeries. Monitoring systems utilize AI for identifying faces and detecting irregularities to improve safety.
Essential Features of AI
AI's distinctive capabilities are what render it a transformative force in various sectors:
Generalized Learning: AI systems adjust to different surroundings and circumstances, much like a robot traversing uncertain landscapes.
Reasoning: AI assesses various alternatives and chooses the optimal path, like determining efficient travel routes.
Problem-Solving: Ranging from intricate computations to tackling real-life challenges, AI is proficient at discovering effective solutions.
Branches of Artificial Intelligence
AI can be divided into two main categories:
Limited AI (Narrow AI):
Concentrated on accomplishing one task effectively. Instances consist of:
AlphaGo: A software created to play and excel in the game of Go.
Alexa: Able to perform multiple tasks within its designed capabilities but lacking genuine comprehension.
Robust AI (General AI):
A conceptual type of AI that demonstrates self-awareness and emotional intelligence, similar to human thought processes. While it is presently theoretical, this could result in machines that can think and make decisions on their own.
AI versus Machine Learning versus Deep Learning
While these terms are frequently utilized synonymously, they indicate distinct elements of intelligent systems:
Artificial Intelligence (AI): The broad domain dedicated to creating smart systems.
Machine Learning (ML): A branch of AI that allows machines to acquire knowledge from data without direct programming.
Deep Learning (DL): A specialized branch of ML, modelled after the neural networks of the human brain, allowing machines to analyse intricate data patterns such as images and audio.
Characteristics | Artificial Intelligence (AI) | Machine Learning (ML) | Deep Learning (DL) |
Definition | Machines that mimic human intelligence | Subset of AI, enables machines to learn from data | Subset of ML, inspired by human brain structure |
Goal | Perform tasks that require human-like intelligence | Learn from data, improve performance | Automatically learn and improve from data |
Approach | Rule-based systems, decision trees | Statistical models, optimization techniques | Neural networks, convolutional networks |
Application | Virtual assistants, expert systems, robotics | Image recognition, natural language processing, recommendation systems | Image classification, speech recognition, natural language processing |
Data Requirements | Structured, unstructured, semi-structured | Labelled, unlabelled | Large datasets, labelled |
Complexity | High-level, abstract | Medium-level, algorithmic | Low-level, computational |
Accuracy | Variable, dependent on programming | High, dependent on data quality | High, dependent on data quality and model complexity |
Training | Programmed rules, decision trees | Supervised, unsupervised, reinforcement learning | Supervised, unsupervised, reinforcement learning |
Scalability | Limited, dependent on programming | Medium, dependent on data size | High, dependent on computational resources |
Interpretability | Low, dependent on programming | Medium, dependent on model complexity | Low, dependent on model complexity |
Key Differences:
AI focuses on human-like intelligence, while ML and DL focus on specific tasks.
ML is a subset of AI, enabling machines to learn from data.
DL is a subset of ML, inspired by human brain structure and function.
Data requirements and complexity vary across AI, ML, and DL.
Real-World Examples:
AI: Virtual assistants (Siri, Alexa), expert systems (medical diagnosis)
ML: Image recognition (Google Photos), natural language processing (chatbots)
DL: Image classification (self-driving cars), speech recognition (voice assistants)
This table provides a concise comparison of AI, Machine Learning, and Deep Learning.
Projections for Artificial Intelligence in the Future
Specialists in technology and innovation have daring forecasts regarding the future of AI:
Ray Kurzweil's Singularity Theory: Kurzweil forecasts that by 2045, AI will achieve a level of intelligence equivalent to that of humans, an occurrence called "the singularity."
Elon Musk’s Aspiration: Musk imagines AI-augmented humans via brain-computer interfaces, fostering a synergistic bond between people and machines.
These predictions suggest a time when human abilities are significantly enhanced by AI, resulting in revolutionary progress across all domains.
Real-World Examples of AI
A few of the most revolutionary AI applications are:
Sophia the Automaton:
Created by Hanson Robotics, Sophia is a humanoid robot recognized for her realistic expressions and has also been awarded citizenship by Saudi Arabia.
Atlas from Boston Dynamics:
This sophisticated robot showcases incredible agility and coordination, able to execute backflips and manoeuvre through rough terrain.
Emotion-Interpreting AI:
These systems analyse human emotions through facial expressions, vocal tone, and body language, creating new opportunities for uses in mental health and customer support.
Challenges and Limitations of AI
Although it holds great promise, AI presents various challenges and ethical issues.
Technical Constraints:
Significant demands for computational power
Reliance on extensive datasets
Problems with network latency
Risks to Privacy and Security:
AI systems frequently need access to confidential information, which raises fears about data leaks and improper use.
Ethical Aspects:
Prejudice and Inequality: AI might inadvertently reinforce biases found in its training datasets.
Job Displacement: Automation could cause a decline in the need for human workers in specific industries.
Legal and Regulatory Obstacles:
Governments are struggling with how to oversee AI, especially regarding intellectual property, accountability, and consumer safety.
The Future of AI: Beyond the Horizon
As AI develops, its uses will grow into new domains, offering possibilities for advancements in:
Medical Care:
AI-powered diagnostic tools and customized medicine have the potential to transform patient care, enabling earlier disease detection and individualized treatment strategies.
Learning:
Virtual tutors and adaptive learning systems offer a more tailored and effective educational experience.
Retail and Online Shopping:
AI-powered immersive shopping experiences could seamlessly integrate the physical and digital retail environments.
Sustainability and Climate Issues:
AI systems are capable of forecasting environmental alterations and improving resource utilization, supporting global sustainability initiatives.
Conclusion
Artificial Intelligence is at the leading edge of technological progress, ready to transform human existence in remarkable ways. Although the path presents difficulties, the possibilities are vast. Transforming industries and improving everyday conveniences, AI serves not merely as a tool but as a driver for a more efficient and improved world.
As we persist in creating and utilizing AI to its maximum capabilities, the future presents limitless opportunities. In healthcare, education, and other fields, AI is set to become an essential partner in creating a more intelligent and interconnected future.
Comentarios